Annotation in Machine Learning: The Key to Data Success
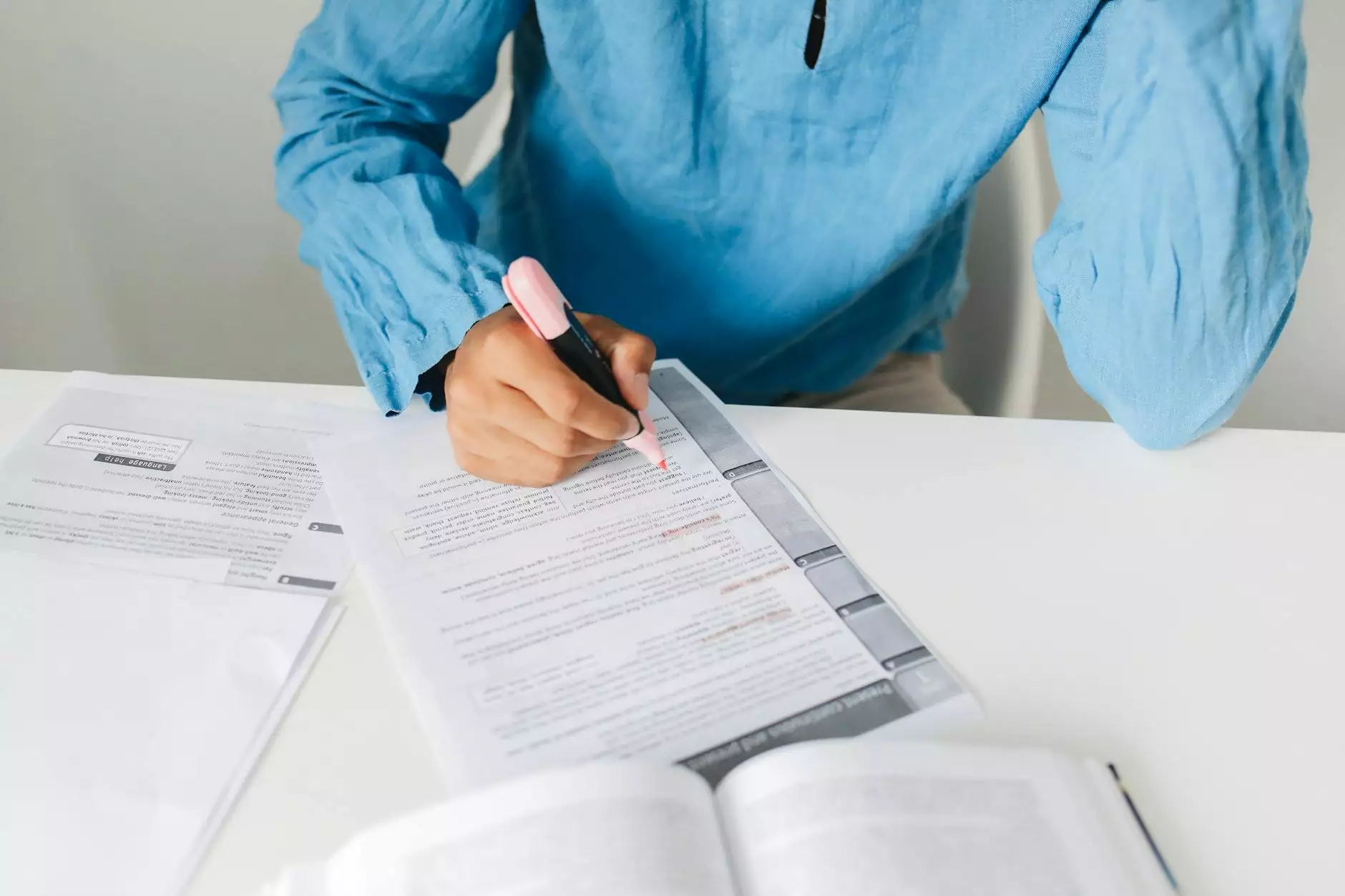
Annotation in machine learning is a critical process that lays the foundation for the development of robust artificial intelligence (AI) systems.
The Importance of Data Annotation
In the realm of machine learning, data is considered the lifeblood of intelligent systems. However, raw data in its initial form is often just a collection of numbers or inputs without much context. This is where data annotation comes into play. By adding meaningful labels to data, we can significantly enhance its usability.
Why is Annotation Critical?
- Enhances Machine Learning Models: Annotated data provides the necessary context for machine learning algorithms to learn from historical examples and make predictions on new data.
- Improves Accuracy: Quality annotations lead to higher accuracy rates in machine learning models, increasing their reliability and effectiveness.
- Facilitates Various Applications: From sentiment analysis to object recognition, annotated data is essential across numerous applications in machine learning.
Types of Annotation in Machine Learning
Understanding the different types of annotation in machine learning can help businesses effectively utilize their data. Here are the primary categories:
1. Text Annotation
Text annotation involves labeling text data to extract meaningful information. Common methods include:
- Named Entity Recognition (NER): Identifies and classifies key elements in the text.
- Sentiment Analysis: Determines the sentiment or emotional tone of the text.
- Text Classification: Categorizes text into predefined groups.
2. Image Annotation
Image annotation is crucial for computer vision tasks, where visual data needs to be interpreted. Annotation methods include:
- Bounding Boxes: Draws rectangles around objects of interest in images.
- Polygon Annotation: Uses precise shapes to identify the exact boundaries of an object.
- Semantic Segmentation: Classifies each pixel in the image into a category.
3. Audio Annotation
For systems that rely on auditory data, audio annotation involves:
- Transcription: Converting spoken words into written text.
- Speaker Identification: Tagging who is speaking in an audio clip.
Challenges of Data Annotation
While annotation is vital, it comes with its own set of challenges. Businesses need to be aware of these to implement effective annotation strategies:
1. Labor-Intensive Process
Annotation can be an arduous task that requires significant human resources, especially with large datasets. This can lead to increased operational costs.
2. Quality Control
Ensuring the quality and accuracy of annotations is paramount. Poor-quality annotations can severely hinder model performance. Hence, a robust quality control mechanism should be in place.
Best Practices for Data Annotation
To overcome these challenges, businesses should adopt best practices to enhance the efficiency and effectiveness of their annotation processes:
1. Use Domain Experts
Involving experts who understand the nuances of the data domain can significantly improve the quality of annotations. Their insights ensure that annotations are both accurate and relevant.
2. Leverage Annotation Tools
Utilizing specialized annotation tools can streamline the process. These tools often come equipped with features that enhance productivity, such as batch processing and quality checks.
3. Continuous Training
Regular training sessions for annotators can keep them up to date with the latest standards and practices, thereby improving the overall quality of the annotations.
Conclusion: The Future of Annotation in Machine Learning
The landscape of machine learning is ever-evolving, and with it, the importance of annotation in machine learning continues to rise. As AI technology advances, the demand for accurate and high-quality annotated data will become even more critical. Businesses that invest in effective annotation strategies today will be well-positioned to reap the benefits of machine learning in the future.
Final Thoughts
In summary, annotation in machine learning acts as the bridge between raw data and intelligent decision-making. It transforms disorganized data into actionable insights that can drive business growth and innovation. By understanding its importance and implementing best practices, businesses can enhance their machine learning initiatives and stay ahead in the competitive landscape.